Research Abstract
バイオ画像の分類のための反復的クラスタリングを用いた能動学習フレームワーク
Active learning framework with iterative clustering for bioimage classification
2012年8月28日 Nature Communications 3 : 1032 doi: 10.1038/ncomms2030
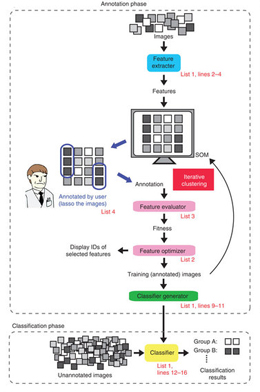
撮像システムの発達により大量の画像が研究現場にもたらされるようになった。この多数の画像を分類するには多大な労力を要するが、分類の半自動化によって労力を軽減することができる。そのために今回新たに私たちが開発した画像分類の仕組み、カルタ(CARTA: Clustering-Aided Rapid Training Agent)について報告する。CARTAは生物医学画像の分類における画像に対するアノテーション工程と画像特徴の選択工程を容易にする、遺伝的アルゴリズムと自己組織化マップを組み合わせた新奇の能動学習アルゴリズムである。CARTAは簡便で対話的なアノテーションと正確な分類精度を提供し、植物やヒト細胞の画像について、細胞内局在や細胞周期、アポトーシスをアノテーター(専門家)と同等かそれ以上に正確なレベルで分類できる。またCARTAは核磁気共鳴画像法(MRI)によるがん細胞の画像や手術後における経時変化を追った多重カラー画像の分類にも適用可能である。加えて、分類のための特徴のカスタマイズ、ハイスループットな表現型解析、そしてユーザーの目的に合ったさまざまな分類基準の採用に対しても、CARTAを適用することができる。
- 東京大学大学院 新領域創成科学研究科 先端生命科学専攻
- 東京理科大学 理工学部 応用生物科学科
- 東京大学大学院 情報理工学系研究科 数理情報学専攻
- 国立がん研究センター東病院 臨床開発センター
Advances in imaging systems have yielded a flood of images into the research field. A semi-automated facility can reduce the laborious task of classifying this large number of images. Here we report the development of a novel framework, CARTA (Clustering-Aided Rapid Training Agent), applicable to bioimage classification that facilitates annotation and selection of features. CARTA comprises an active learning algorithm combined with a genetic algorithm and self-organizing map. The framework provides an easy and interactive annotation method and accurate classification. The CARTA framework enables classification of subcellular localization, mitotic phases and discrimination of apoptosis in images of plant and human cells with an accuracy level greater than or equal to annotators. CARTA can be applied to classification of magnetic resonance imaging of cancer cells or multicolour time-course images after surgery. Furthermore, CARTA can support development of customized features for classification, high-throughput phenotyping and application of various classification schemes dependent on the user's purpose.