Research Abstract
成人の自閉スペクトラム症を少数の異常な脳領域間結合で予測
A small number of abnormal brain connections predicts adult autism spectrum disorder
2016年4月14日 Nature Communications 7 : 11254 doi: 10.1038/ncomms11254
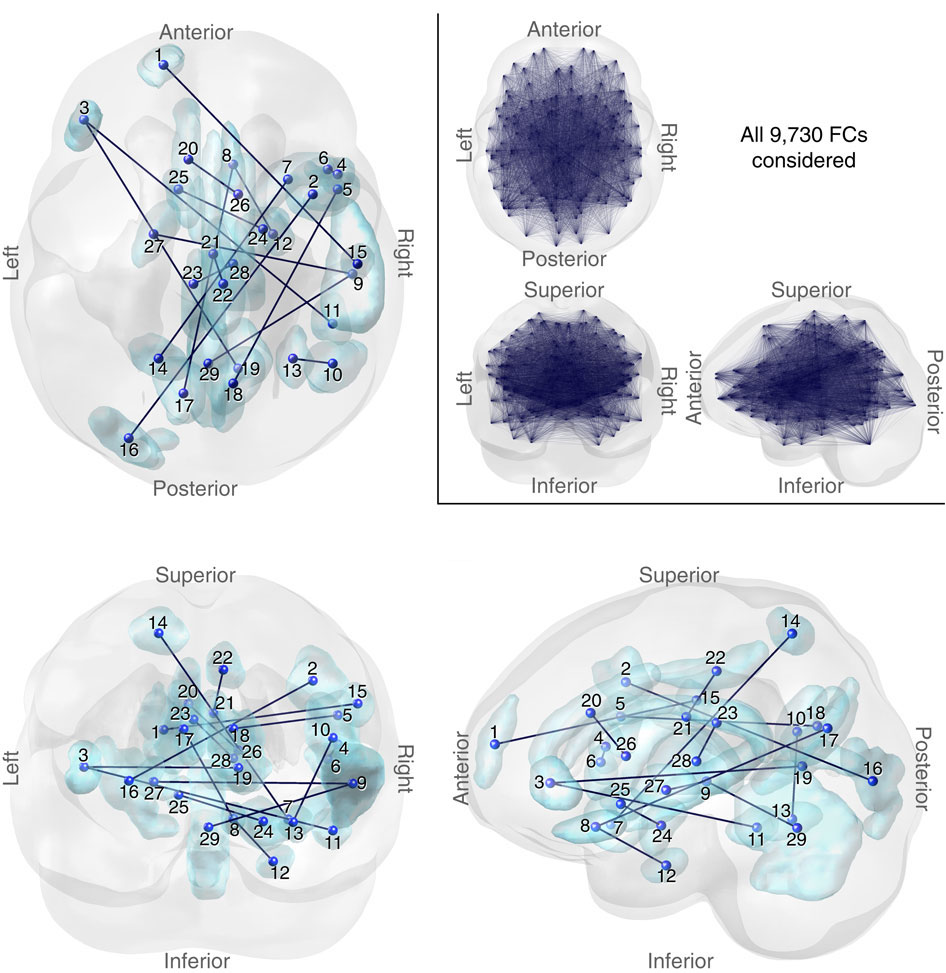
自閉スペクトラム症(ASD)は生涯にわたる深刻な障害だが、その根底にある神経メカニズムはいまだによく分かっていない。近年、神経イメージングに基づいて脳領域間の機能的結合(FC)の異常を見つけだし、ASD者と定型発達(TD)者を判別する方法がいくつか開発された。しかし、過剰適合や、測定条件・人口学的分布の変動がもたらす干渉効果のため、外部のコホートに対する妥当性が厳密に立証された判別法は今のところ皆無である。今回我々は、新規の機械学習アルゴリズムを開発することで、それらの困難を克服し、ASD者とTD者を区別する少数のFCを特定した。完成した判別法は、開発に使った日本人コホートで高い精度を達成し、米国と日本の2つの検証用外部コホートでも顕著な汎化を示した。今回開発したASD判別法は、うつ病患者や注意欠如多動症者を対照群と区別することはできないが、統合失調症患者を対照群とある程度区別することができる。これらの結果は、神経イメージングに基づいて複数の障害スペクトラムを定量化する方法を探ることの実行可能性を示唆するものだ。
Corresponding Authors
Although autism spectrum disorder (ASD) is a serious lifelong condition, its underlying neural mechanism remains unclear. Recently, neuroimaging-based classifiers for ASD and typically developed (TD) individuals were developed to identify the abnormality of functional connections (FCs). Due to over-fitting and interferential effects of varying measurement conditions and demographic distributions, no classifiers have been strictly validated for independent cohorts. Here we overcome these difficulties by developing a novel machine-learning algorithm that identifies a small number of FCs that separates ASD versus TD. The classifier achieves high accuracy for a Japanese discovery cohort and demonstrates a remarkable degree of generalization for two independent validation cohorts in the USA and Japan. The developed ASD classifier does not distinguish individuals with major depressive disorder and attention-deficit hyperactivity disorder from their controls but moderately distinguishes patients with schizophrenia from their controls. The results leave open the viable possibility of exploring neuroimaging-based dimensions quantifying the multiple-disorder spectrum.