人工知能:AIが生成したテキストへの透かし処理
Nature
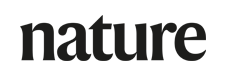
大規模言語モデルによって生成されたテキストにウォーターマーク(電子透かし)を入れることで、合成コンテンツを特定し追跡する能力を向上させるツールを報告する論文が、今週のNatureに掲載される。
大規模言語モデル(LLM:Large language models)は、チャットボットや執筆支援など、さまざまな用途でテキストを生成できる人工知能(AI:artificial intelligence)ツールとして広く使用されている。しかし、AIが生成したテキストを特定の生成元に帰属させることは難しく、情報の信頼性が疑問視されることもある。この問題の解決策として透かしが提案されているが、実用システムでは品質と計算効率に関する厳しい要件があるため、大規模に展開されていない。
Sumanth DathathriおよびPushmeet Kohliらは、AI生成テキストに透かしを適用する新しいサンプリングアルゴリズムを使用する手法として知られるSynthID-Textを開発した。このツールは、サンプリングアルゴリズムを使用してLLMの単語選択に微妙な偏りを加え、関連する検出ソフトウェアによって認識可能な署名を挿入する。これは、「歪曲」モードを通じて行うことができ、この場合は出力品質を若干犠牲にして透かしを改善する。あるいは、「非歪曲」モードを通じて行うこともでき、この場合はテキストの品質を維持する。
これらの透かし文字の検出可能性は、一般に利用可能な複数のモデルで評価され、SynthID-Textは既存の方法と比較して検出可能性が向上していることが示された。テキストの品質も、Gemini LLMを使用したライブチャットのやりとりから得られた約2,000万件の回答を使用して評価され、その結果、透かし文字の非歪曲モードではテキストの品質が低下しないことが示唆された。さらに、SynthID-Textの使用は、LLMの実行に必要な演算能力への影響はごくわずかであり、実装への障壁を低減する。
著者らは、テキストの透かしは出力の編集や言い換えによって回避できる可能性があると警告している。しかし、この研究は、AI生成コンテンツに対して生成テキストの透かしを生成できるツールの実用性を示しており、LLMの責任ある利用における説明責任と透明性をさらに向上させるためのさらなる一歩である。
Dathathri, S., See, A., Ghaisas, S. et al. Scalable watermarking for identifying large language model outputs. Nature 634, 818–823 (2024). https://doi.org/10.1038/s41586-024-08025-4
A tool that can watermark text generated by large language models, improving the ability for it to identify and trace synthetic content, is described in Nature this week.
Large language models (LLMs) are widely used artificial intelligence (AI) tools that can generate text for chatbots, writing support and other purposes. However, it can be difficult to identify and attribute AI-generated text to a specific source, putting the reliability of the information into question. Watermarks have been proposed as a solution to this problem, but have not been deployed at scale because of stringent quality and computational efficiency requirements in production systems.
Sumanth Dathathri, Pushmeet Kohli and colleagues developed a scheme that uses a novel sampling algorithm to apply watermarks to AI-generated text, known as SynthID-Text. The tool uses a sampling algorithm to subtly bias the word choice of the LLM, inserting a signature that can be recognized by the associated detection software. This can either be done via a ‘distortionary’ pathway, which improves the watermark at a slight cost of output quality, or a ‘non-distortionary’ pathway, which preserves text quality.
The detectability of these watermarks was evaluated across several publicly available models, with SynthID-Text showing improved detectability compared to existing approaches. The quality of the text was also assessed using nearly 20 million responses from live chat interactions using the Gemini LLM, with results suggesting that the non-distortionary mode of watermarking did not decrease the text quality. Finally, the use of SynthID-Text has a negligible impact on the computational power needed to run the LLM, reducing the barrier to implementation.
The authors caution that text watermarks can be circumvented by editing or paraphrasing the output. However, this work shows viability for a tool that can produce generative text watermarks for AI-generated content, in a further step to improving the accountability and transparency of responsible LLM use.
doi: 10.1038/s41586-024-08025-4
「Nature 関連誌注目のハイライト」は、ネイチャー広報部門が報道関係者向けに作成したリリースを翻訳したものです。より正確かつ詳細な情報が必要な場合には、必ず原著論文をご覧ください。