【人工知能】ロンドン地下鉄を使いこなす機械学習モデル
Nature
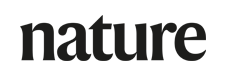
神経ネットワークとコンピューターの最も優れた特徴を組み合わせたハイブリッド型機械学習モデルについて記述された論文が、今週掲載される。
従来のコンピューターは、複雑な形式のデータを処理できるが、そうした課題を実行するには人手によるプログラミングを必要とする。これに対して人工ニューラルネットワーク(人工神経回路網)は、データ中にパターンを見つけ出す能力を有する脳のような学習を模倣するように開発されたが、構造化データの記号処理に必要なメモリアーキテクチャーがない。
今回、Alex Graves、Greg Wayne、Demis Hassabisたちの研究グループは、いわゆる“Differentiable Neural Computer (DNC)”を開発した。これは、事例からの学習や試行錯誤による学習ができるニューラルネットワークと従来のコンピューターのランダムアクセスメモリーに似た外部記憶構造によって構成されている。そのため、DNCは、ニューラルネットワークのように学習できる一方で、コンピューターのように複雑なデータの処理も可能だ。
今回の研究は、DNCが、家系図や交通ネットワークのようなグラフ構造を理解でき、例えば、予備知識なしにロンドン地下鉄における最適な乗り継ぎ経路を導き出し、目的地が記号言語で記述された移動ブロックパズルを解けることを明らかにしている。
A hybrid learning machine that combines the best features of neural networks and computers is described in a study published in Nature this week.
Conventional computers can process complex forms of data, but require manual programming to perform these tasks. Artificial neural networks have been developed to mimic brain-like learning that can identify patterns in data, but they lack the memory architectures needed for symbolic processing of structured data.
Alex Graves, Greg Wayne, Demis Hassabis and colleagues developed a so-called ‘differentiable neural computer’ (DNC), which comprises a neural network that can learn by example or through trial and error, and an external memory structure similar to random-access memory in a conventional computer. Thus, it can learn like a neural network but process complicated data like a computer.
The study shows the DNC can successfully understand graph structures like family trees or transport networks; for example, planning the best route on the London Underground without prior knowledge of this transport system or solving moving block puzzles with goals described in a symbolic language.
doi: 10.1038/nature20101
「Nature 関連誌注目のハイライト」は、ネイチャー広報部門が報道関係者向けに作成したリリースを翻訳したものです。より正確かつ詳細な情報が必要な場合には、必ず原著論文をご覧ください。