がん:機械学習の手法で肺がん検診を改善できるかもしれない
Nature
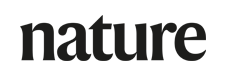
早期肺がん患者を特定できる機械学習法について報告する論文が、今週Nature に掲載される。この機械学習の手法によって血液検体中の腫瘍由来DNAが検出されるので(いわゆる「液体生検」)、肺がんリスクの高い者がもっと頻繁に検診を受けられるようにするために役立つ可能性がある。
肺がんリスクの高い者に推奨される検診方法はCTスキャンであり、これによって肺がん関連死が減ったことが明らかになっている。しかし、CTスキャンは、費用が高いこと、検診プログラムが少ないこと、偽陽性に対する懸念などの要因により、受診率が低迷しており、米国では適格者の約5%しか受診していない。CTスキャンに代わる魅力的な肺がん検診方法が血液検査だが、ほとんどの液体生検研究は、進行肺がん患者のモニタリングに着目したものになっており、進行肺がん患者の腫瘍関連DNAマーカーの濃度は、早期肺がん患者よりも高い可能性がある。
今回、Maximilian Diehnたちの研究チームは、循環血中腫瘍DNA(ctDNA)を評価するために既存の塩基配列決定法を最適化した。その結果、DNAの回収が改善され、肺がんの有用なマーカーとして役立つ可能性のあるDNAの変化が同定された。また、Diehnたちは、この塩基配列決定法を用いて、早期肺がんにおけるctDNAの値は非常に低いが、ctDNAが早期肺がんの強力な予後マーカーであることを明らかにした。さらにDiehnたちは、これらのデータを利用して、血液検体中の肺がん由来DNAの存在を予測するための機械学習法を改良した。小細胞肺がん以外の早期肺がん患者104人とそれにマッチした対照群56人の初回検体を用いて、この方法を検証したところ、早期肺がん患者とそれにリスクがマッチした対照群の患者とを区別できた。この結果は、早期肺がん患者群46人と対照群48人からなる独立検証コホートにおいて確認された。
A machine-learning method that can identify patients with early-stage lung cancer is described in Nature this week. The approach detects tumour-derived DNA in blood samples — so-called liquid biopsies — and may help to increase the adoption of screening for high-risk individuals.
The recommended mode of screening for lung cancer in high-risk individuals is CT scanning, which has been shown to reduce lung cancer-related deaths. However, uptake is low owing to factors such as high costs, limited screening programmes and concerns with false positives; around 5% of eligible individuals undergo such screening in the United States. Blood tests are an attractive alternative means of cancer detection, although most liquid biopsy studies focus on monitoring patients with advanced disease, who may have higher levels of tumour-related DNA markers than early-stage patients.
Maximilian Diehn and colleagues optimize an existing sequencing method for assessing circulating tumour DNA (ctDNA). They improve the recovery of DNA and identify alterations that may serve as useful markers of disease. Using this method the authors show that although ctDNA is present in only very low levels in early-stage lung cancers, it is a strong prognostic marker. They go on to use these data to refine a machine-learning method for predicting the presence of lung-cancer-derived DNA in blood samples. This technique discriminates early-stage lung cancer patients from risk-matched controls in an initial sample of 104 patients with early stage non-small cell lung cancer and 56 matched controls, which was confirmed in an independent validation cohort of 46 cases and 48 controls.
doi: 10.1038/s41586-020-2140-0
「Nature 関連誌注目のハイライト」は、ネイチャー広報部門が報道関係者向けに作成したリリースを翻訳したものです。より正確かつ詳細な情報が必要な場合には、必ず原著論文をご覧ください。