計算機科学:人工知能を利用して効率の高いアルゴリズムを発見する
Nature
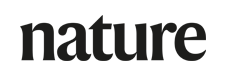
ディープマインド社が考案した深層強化学習法を用いた行列乗算アルゴリズムの自動発見について報告するAlhussein Fawziたちの論文が、今週、Nature に掲載される。この深層強化学習法では、現在最もよく知られているアルゴリズムだけでなく、人間やコンピューターが設計した従来のアルゴリズムよりも高速な新しいアルゴリズムが迅速に再発見される。この研究知見は、既存の計算タスクをもっと効率的に解く方法を発見するという深層強化学習法の可能性を浮き彫りにしている。
基礎的な計算操作を実行するアルゴリズムの効率が向上すると、数多くの計算の全体的な速度が影響を受けることがある。この論文では、重要な原始的な計算タスクである行列乗算のアルゴリズムを自動発見するための深層強化学習法について説明されている。行列乗算は、膨大な量の計算において日常的に使用されている。このシステムは、AlphaTensorと名付けられ、2つの行列(数字の配列)を乗算する最適な方法を発見することを目的としたゲームをプレイするという課題が与えられている。このゲームは、伝統的なゲーム(チェス、囲碁など)よりもはるかに難しく、場合によってはアクションの回数が約1兆回も多く必要とされる。AlphaTensorは、既知のアルゴリズムを特定し、それによってシステムが機能することを証明しただけでなく、全く新しいアルゴリズムも発見した。また、こうしたアルゴリズムの発見によって、50年以上にわたって相当量の研究が行われたにもかかわらず改良されることのなかったアルゴリズムの改良も実現した。さらにAlphaTensorを最適化することで、特定の種類のコンピューター上で特定の状況下で特に有効に動作するアルゴリズムを発見できるかもしれない。
Fawziたちは、AlphaTensorのいくつかの限界も指摘している。例えば、AlphaTensorが必要とする事前定義されたコンポーネントの一部のために効率的なアルゴリズムの一部が見逃されてしまう可能性があるのだ。しかし、AlphaTensorによって発見されるアルゴリズムは、乗算アルゴリズムを使用する計算タスクを改良できるだけでなく、強化学習を使用して既知の問題に対する予想外の新しい解法を発見する過程を示せるかもしれない。
The automated discovery of algorithms for multiplication of matrices, using a deep reinforcement learning approach devised by DeepMind, is reported in Nature this week. This approach quickly re-discovers the best currently known algorithms, but also new ones that are faster than any previous human and computer-designed algorithms. The findings highlight the potential of deep reinforcement learning for finding new approaches for solving more efficiently existing computational tasks.
Improving the efficiency of algorithms that execute fundamental computational operations can affect the overall speed of a large number of computations. Alhussein Fawzi and colleagues describe a deep reinforcement learning approach for the automated discovery of algorithms for an important primitive computational task: matrix multiplication, which is routinely used in vast arrays of computations. The system, named AlphaTensor, is tasked with playing a game in which the goal is to find the best way to multiply two matrices (arrays of numbers). This game is far more challenging than traditional games (such as chess or Go), requiring around one trillion more actions in some cases. AlphaTensor identifies previously known algorithms (thereby proving the system works) as well as finding completely new algorithms. In some cases, the discoveries improve upon algorithms that haven’t been improved for more than 50 years despite much research. Furthermore, AlphaTensor could be optimized to discover algorithms that work particularly well in certain circumstances, for running on specific types of computers.
The authors note some limitations to their system, such as it needing some predefined components that may cause it to miss a subset of efficient algorithms. However, AlphaTensor’s discoveries could improve computational tasks that use multiplication algorithms as well as demonstrate how reinforcement learning can be used to find new and unexpected solutions to known problems.
doi: 10.1038/s41586-022-05172-4
「Nature 関連誌注目のハイライト」は、ネイチャー広報部門が報道関係者向けに作成したリリースを翻訳したものです。より正確かつ詳細な情報が必要な場合には、必ず原著論文をご覧ください。