環境:機械学習による気象と気候予測の改善
Nature
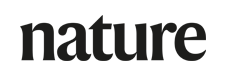
正確な気象予測と気候シミュレーションの両方が可能な機械学習モデルに関する論文が、今週のNatureに掲載される。NeuralGCMと名付けられたこのモデルは、既存のいくつかの気象と気候予測モデルを凌駕し、従来のモデルよりも計算力を大幅に節約できる可能性を秘めている。
大循環モデル(GCM;General circulation models)は、大気、海洋、陸地の物理的な過程を表すもので、気象と気候予測の基礎となっている。長期予測にまつわる不確実性を減らし、異常気象を推定することは、気候緩和と適応を理解するための鍵となる。機械学習モデルは、計算コストを削減できるという利点があり、気象予測の代替手段として提案されてきたが、長期予測に関しては、GCMほど性能が高くないことが多い。
Stephan Hoyerらは、機械学習と物理学に基づく手法を組み合わせたモデル、NeuralGCMを設計した。このモデルは、短期および中期の気象予報を行うだけでなく、数十年にわたる気候のシミュレーションも行うことができる。このモデルは、1-15日先までの予報では、欧州中期気象予報センター(ECMWF;European Centre for Medium-Range Weather Forecasts、従来の物理学に基づく気象モデルの中で最も優れたもののひとつ)の予報精度に匹敵する。10日先までの予報では、NeuralGCMは既存の機械学習アプローチの精度と匹敵し、時にはそれを上回る。
NeuralGCMは、最高の機械学習や物理学を基にした手法と同レベルの精度で気候シミュレーションを行う。著者らは、NeuralGCMを使用して40年間の気候予測に海面の水温を含めたところ、このモデルが出した結果は、ECMWFのデータに見られる地球温暖化の傾向と一致することを発見した。NeuralGCMは、サイクロンとその軌道の予測においても、既存の気候モデルを上回った。これらの結果は、機械学習がGCMを改善するための有効な手段であることを示唆している、と著者らは結論づけている。
Kochkov, D., Yuval, J., Langmore, I. et al. Neural general circulation models for weather and climate. Nature (2024).
A machine learning model capable of both accurate weather predictions and climate simulations is presented in Nature this week. The model, named NeuralGCM, outperforms some existing weather and climate prediction models and has the potential to make large savings in computational power over conventional models.
General circulation models (GCMs), representing physical processes of the atmosphere, ocean and land, are the basis for weather and climate predictions. Reducing the uncertainty around long-term forecasting and estimating extreme weather events are key to helping understand climate mitigation and adaptation. Machine learning models have been suggested as an alternative approach to weather prediction with the benefit of reduced computational costs, but they often do not perform as well as GCMs when it comes to long-term forecasting.
Stephan Hoyer and colleagues designed NeuralGCM, a model that combines machine learning and physics-based methods, which can make short- and medium-range weather forecasts as well as simulating climate over a number of decades. The model can compete with the accuracy of the European Centre for Medium-Range Weather Forecasts (ECMWF, one of the best conventional physics-based weather models) predictions for 1–15-day forecasts. For forecasts up to 10 days in advance, NeuralGCM competes with and sometimes exceeds the accuracy of existing machine learning approaches.
NeuralGCM produces climate simulations at the same level of accuracy as the best machine learning and physics-based methods. When the authors included sea surface temperatures in 40-year climate predictions using NeuralGCM, they found that the outcomes the model produced mapped with the global warming trends seen in data from the ECMWF. NeuralGCM also outperformed pre-existing climate models in predicting cyclones and their trajectories. Together, these findings suggest that machine learning is a viable approach for improving GCMs, the authors conclude.
doi: 10.1038/s41586-024-07744-y
「Nature 関連誌注目のハイライト」は、ネイチャー広報部門が報道関係者向けに作成したリリースを翻訳したものです。より正確かつ詳細な情報が必要な場合には、必ず原著論文をご覧ください。