コンピューターサイエンス:生成AIのデータで訓練されたAIモデルが崩壊する可能性
Nature
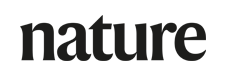
AIが生成したデータセットを次世代の機械学習モデルの学習に使用すると、その出力が汚染される可能性があることを報告する論文が、Natureに掲載される。この研究は、数世代以内にオリジナルのコンテンツが無関係のナンセンスなものに置き換えられてしまうことを示しており、AIモデルの学習に信頼性の高いデータを使用することの重要性を示している。
大規模言語モデル(LLMs;Large Language Models)のような生成AIツールの人気は高まっており、主に人間が生成した入力を使って訓練されてきた。しかし、これらのAIモデルがインターネット上で普及し続けるにつれ、コンピュータが生成したコンテンツが、再帰的ループの中で他のAIモデル、あるいは自分自身を訓練するために使用される可能性がある。
Ilia Shumailovらは、AIモデルがどのようにモデル崩壊を起こすかを説明する数学モデルを提示している。著者らは、AIが学習データ内の特定の出力(例えば、あまり一般的でないテキストの行)を見落とし、データセットの一部のみでAI自身を学習させる可能性があることを実証している。Shumailovらは、人工知能で作成された訓練データセットに対するAIモデルの反応についても調査した。その結果、AIが生成したデータをモデルに与えると、世代が進むにつれて学習能力が低下し、最終的にはモデルが崩壊することがわかった。著者らがテストした再帰的に訓練された言語モデルのほぼすべてが、繰り返しのフレーズを表示する傾向があった。例えば、中世の建築物に関するテキストを入力としてテストを行ったところ、第9世代までに出力されたのはジャックラビット(野生のうさぎ)のリストだった。
著者らは、モデルの崩壊は、前の世代が作成した学習データセットを使用するAIモデルの必然的な結果であると提案している。Shumailovらは、人工知能を自らの出力でうまく訓練するために、AIが生成したデータでモデルを訓練することは不可能ではないが、そのデータのフィルタリングには真剣に取り組まなければならないと提案している。同時に、人間が生成したコンテンツに依存しているテック企業は、競合他社よりも効果的なAIモデルを訓練できるかもしれない。
Shumailov, I., Shumaylov, Z., Zhao, Y. et al. AI models collapse when trained on recursively generated data. Nature 631, 755–759 (2024).
Using AI-generated datasets to train future generations of machine learning models may pollute their output, a concept known as model collapse, according to a paper published in Nature. The research shows that within a few generations, original content is replaced by unrelated nonsense, demonstrating the importance of using reliable data to train AI models.
Generative AI tools such as large language models (LLMs) have grown in popularity and have been primarily trained using human-generated inputs. However, as these AI models continue to proliferate across the Internet, computer-generated content may be used to train other AI models — or themselves — in a recursive loop.
Ilia Shumailov and colleagues present mathematical models to illustrate how AI models may experience model collapse. The authors demonstrate that an AI may overlook certain outputs (for example, less common lines of text) in training data, causing it to train itself on only a portion of the dataset. Shumailov and colleagues also investigated how AI models responded to a training dataset that was predominantly created with artificial intelligence. They found that feeding a model AI-generated data causes proceeding generations to degrade in their ability to learn, eventually leading to model collapse. Nearly all of the recursively trained language models they tested tended to display repeating phrases. For example, a test was run using text about medieval architecture as the original input and by the ninth generation the output was a list of jackrabbits.
The authors propose that model collapse is an inevitable outcome of AI models that use training datasets created by previous generations. In order to successfully train artificial intelligence with its own outputs, Shumailov and colleagues suggest that training a model with AI-generated data is not impossible, but the filtering of that data must be taken seriously. At the same time, tech firms that rely on human-generated content may be able to train AI models that are more effective over their competitors.
doi: 10.1038/s41586-024-07566-y
「Nature 関連誌注目のハイライト」は、ネイチャー広報部門が報道関係者向けに作成したリリースを翻訳したものです。より正確かつ詳細な情報が必要な場合には、必ず原著論文をご覧ください。